Understanding the Basics About Machine Learning
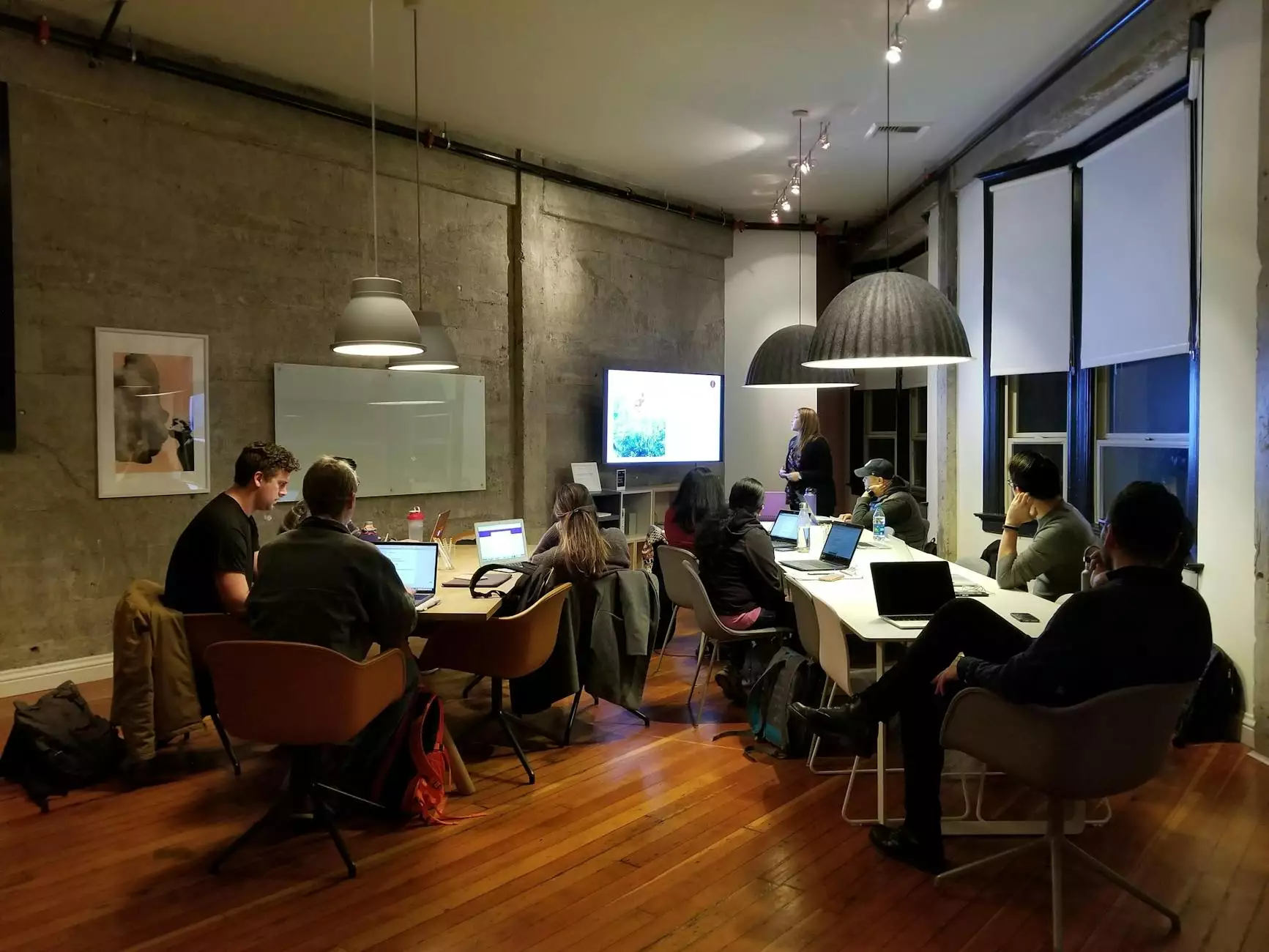
Machine learning is a rapidly evolving field that has gained immense popularity in recent years. As businesses worldwide strive to leverage technology, understanding the basics about machine learning has become increasingly important. In this comprehensive article, we will explore what machine learning is, its historical development, how it operates, its various types, and its profound impact on today’s business landscape.
The Fundamentals of Machine Learning
At its core, machine learning is a subset of artificial intelligence (AI) that allows computers to learn from data and make decisions without being explicitly programmed. This technology enables systems to improve their performance as they are exposed to more data over time.
A Brief History of Machine Learning
The concept of machine learning dates back to the mid-20th century, with pioneering work by famous researchers like Alan Turing and Arthur Samuel. Samuel defined machine learning as a field of study that gives computers the ability to learn without being programmed explicitly. In recent years, advancements in computing power, algorithms, and the availability of vast amounts of data have spurred a massive growth in machine learning applications.
How Does Machine Learning Work?
The essence of machine learning is to identify patterns in data. Here’s a step-by-step breakdown of how the process generally works:
- Data Collection: The first step involves gathering relevant data. This data can come from various sources such as databases, online transactions, social media, and sensor data.
- Data Preprocessing: Once data is collected, it must be cleaned and organized. This step involves handling missing values, removing duplicates, and normalizing data to ensure accurate results.
- Choosing a Model: After preprocessing, the next step is selecting a suitable machine learning model. Various algorithms, such as regression, decision trees, and neural networks, can be employed depending on your specific use case.
- Training the Model: The model is trained on a dataset where it learns the relationship between the input and output variables. This training involves adjusting parameters to minimize errors in predictions.
- Evaluation: After training, the model is evaluated using a separate dataset to test its accuracy and performance. Metrics like precision, recall, and F1-score are often used to measure success.
- Deployment: Once satisfied with the model’s performance, it gets deployed in a real-world environment where it can start making predictions or decisions based on new data.
Types of Machine Learning
Machine learning can be categorized into three main types, each with its unique techniques and applications:
1. Supervised Learning
Supervised learning is the most prevalent type, where the model is trained on labeled data. This means the input data comes with corresponding output labels, allowing the model to learn from this relationship. Typical applications include:
- Spam Detection
- Sentiment Analysis
- Image Classification
2. Unsupervised Learning
Unlike supervised learning, unsupervised learning works with unlabeled data. The model must identify hidden patterns or intrinsic structures in the data. Common applications include:
- Customer Segmentation
- Market Basket Analysis
- Anomaly Detection
3. Reinforcement Learning
In reinforcement learning, an agent learns to make decisions by trying actions in an environment to maximize cumulative rewards. This category is widely used in:
- Game Playing
- Robotics
- Autonomous Vehicles
Applications of Machine Learning in Business
The applications of machine learning in business are vast and transformative. Here are some key areas where machine learning is making a significant impact:
1. Predictive Analytics
Predictive analytics utilizes machine learning algorithms to analyze historical data and forecast future events. Businesses can leverage these insights for:
- Inventory management
- Sales forecasting
- Customer behavior prediction
2. Customer Service Automation
Machine learning enhances customer service through chatbots and virtual assistants. These systems can:
- Respond to queries instantly
- Analyze customer sentiment
- Provide personalized recommendations
3. Fraud Detection
In finance, machine learning algorithms can identify fraudulent transactions by detecting patterns and anomalies in transaction data. This capability allows businesses to:
- Reduce losses from fraud
- Improve compliance with regulations
- Enhance overall security
4. Marketing Optimization
Companies are utilizing machine learning to optimize their marketing strategies by analyzing customer data and assessing campaign effectiveness. This helps them:
- Create targeted marketing campaigns
- Enhance customer engagement
- Increase ROI on marketing efforts
The Challenges of Implementing Machine Learning
While machine learning offers numerous benefits, businesses also face several challenges when implementing these technologies:
1. Data Quality and Availability
The effectiveness of machine learning algorithms heavily depends on the quality and breadth of the data available. Poor quality data can lead to inaccurate models.
2. Talent Shortage
There is a significant demand for skilled machine learning professionals. Organizations often struggle to find the right talent to develop and maintain machine learning systems.
3. Ethical Considerations
As machine learning systems can inadvertently perpetuate biases present in training data, businesses must navigate ethical considerations surrounding fairness and accountability in AI.
4. Integrating Machine Learning into Existing Systems
Integrating machine learning solutions into legacy systems can be complex and resource-intensive, presenting another hurdle for many businesses.
The Future of Machine Learning
As we look ahead, the future of machine learning appears promising. Key trends likely to shape its evolution include:
1. Increased Automation
Machine learning will continue to automate repetitive tasks, allowing employees to focus on strategic initiatives. This will enhance efficiency and productivity across various sectors.
2. Growth of AI Ethics
With growing concerns about data privacy and algorithmic bias, organizations will increasingly prioritize ethical guidelines in developing and deploying machine learning technologies.
3. Rise of Explainable AI
As machine learning systems become more ubiquitous, the demand for explainable AI will increase. Stakeholders will want to understand how models make decisions and predictions.
4. Enhanced Personalization
Businesses will harness the power of machine learning to provide even more personalized customer experiences, analyzing user behavior to tailor products and services effectively.
Conclusion
Understanding the basics about machine learning is essential for anyone in the business arena today. The transformative potential of machine learning offers businesses the ability to enhance their operations, improve customer satisfaction, and drive growth. As technology continues to evolve, staying informed and adept in machine learning will be crucial for ongoing success. By embracing these technologies, businesses can not only stay competitive but also pave the way for innovation and efficiency.