Unlocking the Power of Machine Learning Data Labeling
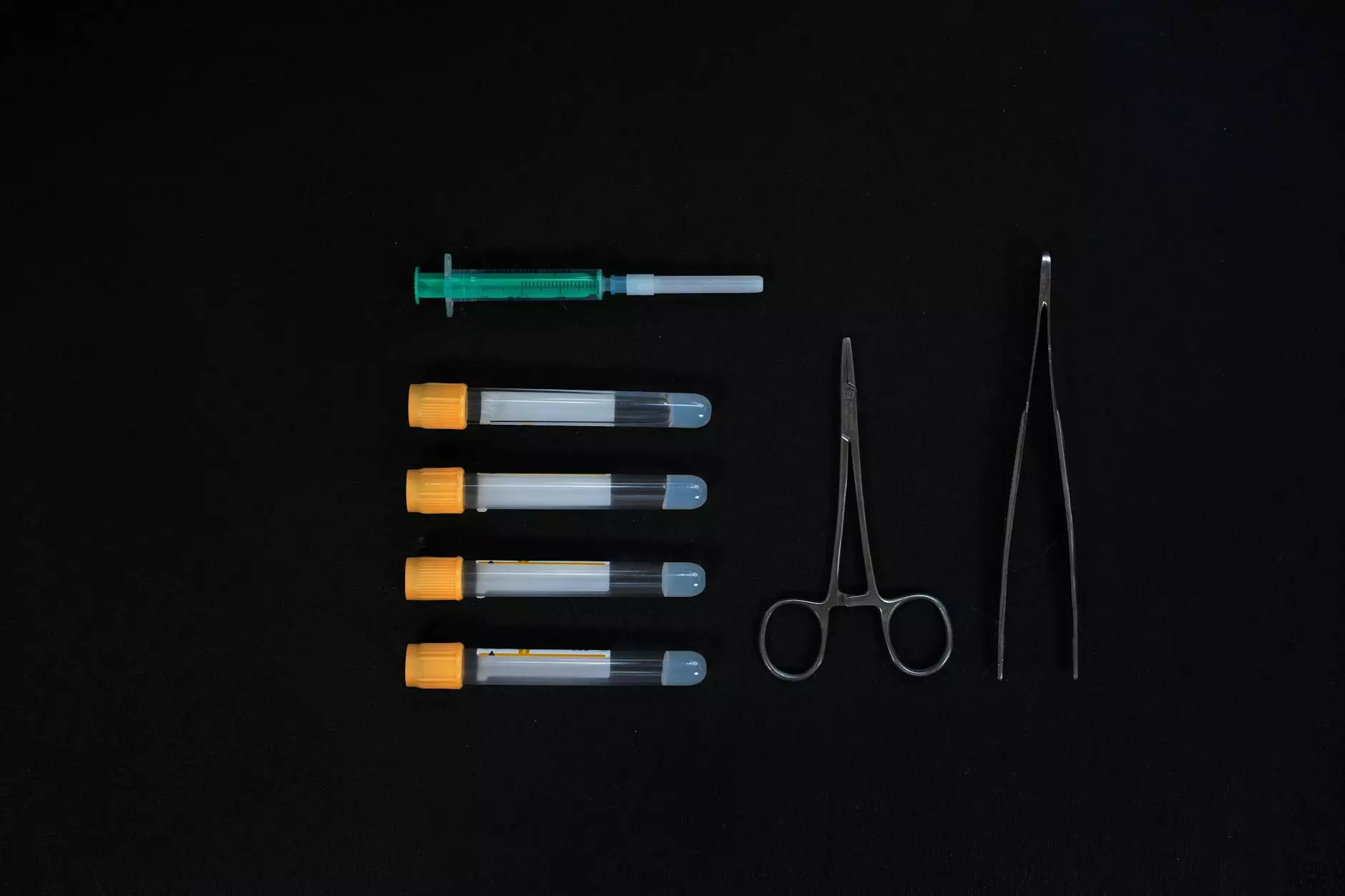
Machine learning data labeling is evolving into a critical component of successful AI and machine learning projects. As companies increasingly rely on AI to drive business decisions, the importance of accurate and efficient data labeling cannot be overstated. This comprehensive guide will explore the intricacies of data labeling, the various tools available, and its impact on machine learning and artificial intelligence.
The Importance of Data Labeling in Machine Learning
Data labeling is the process of categorizing and annotating data so that machine learning models can learn from it. As machine learning algorithms excel at detecting patterns within data, they require meticulously labeled datasets to perform effectively. In essence, data labeling serves as the foundation upon which machine learning models are built. Without high-quality labeled data, even the most advanced algorithms will fail to deliver accurate predictions.
Why Machine Learning Needs Data Labeling
- Training Accuracy: Labeled data significantly enhances the accuracy of machine learning models by providing clear examples of input-output relationships.
- Model Improvement: Iteratively labeled datasets enable models to learn continuously, improving their performance over time.
- Error Reduction: Well-labeled data reduces the occurrence of bias and errors in model predictions, creating more reliable AI systems.
Different Types of Data Labeling
Understanding various data labeling methodologies is crucial for organizations aiming to implement effective machine learning solutions. Here are the common types of data labeling:
1. Image Annotation
Image annotation refers to the process of labeling images for computer vision tasks. Techniques include:
- Bounding Boxes: Drawing boxes around objects within an image to identify them.
- Segmentation: Classifying each pixel of an image to delineate objects more accurately.
- Keypoint Annotation: Identifying specific points on objects, useful for posture detection and facial recognition.
2. Text Annotation
Text annotation involves labeling data in textual format, including:
- Sentiment Analysis: Marking text as positive, negative, or neutral.
- Named Entity Recognition (NER): Identifying entities such as people, organizations, and locations within text.
- Text Classification: Categorizing documents into predefined classes.
3. Audio Annotation
This involves labeling audio data for various applications, including:
- Speech Recognition: Transcribing audio to text and identifying speakers.
- Sound Classification: Labeling sounds by their type (e.g., speech, music, noise).
The Machine Learning Data Labeling Process
The process of machine learning data labeling typically follows several stages:
1. Data Collection
Gathering data from various sources, including public datasets, company databases, and user-generated content, is the first step.
2. Annotation Guidelines Development
Establishing clear guidelines ensures consistency and quality in labeling processes.
3. Labeling
This critical stage involves applying the developed guidelines to categorize and annotate the data. It can be performed manually or through automated tools.
4. Quality Control
Implementing a quality assurance process to review and validate the labeled data is vital. Techniques include reviews by data experts and cross-validation with other annotations.
5. Iteration
Continuous feedback and refinements help improve both the labeling process and the machine learning models.
Tools for Machine Learning Data Labeling
To optimize the data labeling process, various tools are available, catering to different data types and project requirements. Here’s an overview of some popular tools:
- KeyLabs AI: A comprehensive data annotation platform that offers intuitive and customizable solutions for various annotation tasks.
- Labelbox: A scalable data labeling platform that combines human intelligence with machine learning for improved accuracy.
- Alegion: Provides tools for hiring and managing annotators while guaranteeing quality control.
- SuperAnnotate: An image and video annotation tool that focuses on efficiency and workflow automation.
Benefits of Machine Learning Data Labeling
Investing time and resources into data labeling translates into several tangible benefits for businesses and projects. The essential benefits include:
1. Enhanced Model Performance
Accurate labeling leads to better training and, consequently, improved performance when models are deployed. This means more reliable outputs in real-world applications.
2. Faster Time to Market
With the right tools and processes, organizations can speed up the data labeling phase, leading to quicker deployment of machine learning solutions.
3. Scalability
Automated labeling solutions and advanced annotation tools allow businesses to handle larger datasets efficiently, ensuring scalability as data needs grow.
4. Cost Efficiency
Though initial costs may be evident, investing in high-quality data labeling ultimately reduces long-term operational costs by ensuring the model’s success and reducing the need for extensive retraining.
Challenges in Machine Learning Data Labeling
Despite its many benefits, data labeling is fraught with challenges. Recognizing these can help businesses navigate the landscape more effectively:
1. Maintaining Consistency
Ensuring all labeled data adheres to the same quality standards is a common challenge, especially with large teams involved in manual labeling.
2. Data Bias
If the data labeling process is not well-controlled, it can introduce biases that affect model performance, leading to skewed results.
3. Scalability of Manual Processes
While manual processes may provide high accuracy, they are often slow and hard to scale. Businesses must consider automation to handle increasing volumes of data efficiently.
4. Resource Intensive
Labeling data is often time-consuming and requires skilled personnel, which can be a considerable investment.
Future Trends in Machine Learning Data Labeling
As the field of machine learning continues to evolve, so does data labeling. Here are some rising trends:
1. Automation and Artificial Intelligence
Machine learning and AI are increasingly being integrated into data labeling processes, allowing for automated suggestions and quality checks.
2. Crowdsourcing
Leveraging large online communities for data labeling is becoming more prevalent, helping expedite the process and reduce costs.
3. Real-time Labeling
Emerging technologies enable real-time data labeling, significantly reducing latency in feedback loops and improving operational efficiency.
Conclusion: Embracing the Future of Data Annotation
As businesses seek to harness the full potential of machine learning, machine learning data labeling stands out as a critical element for success. By investing in efficient processes, employing the right tools, and ensuring high-quality labeled data, organizations can unlock valuable insights and drive innovation in their fields.
Explore the comprehensive solutions offered by KeyLabs AI that cater to your data annotation needs. As the demand for well-labeled data continues to grow, become a leader in adopting cutting-edge practices for data labeling and annotation.